The mainstream approach to self-driving cars has hit limitations, but a new wave of startups believes that advanced AI can help autonomous vehicles adapt to new roads faster than ever.
AI-Driven Approach to Self-Driving
Four years ago, Wayve’s CEO, Alex Kendall, experimented with a unique AI approach to teach a car to drive using only a few cameras and a large neural network. The car initially struggled on a small country road in the UK but quickly improved. Using reinforcement learning, the AI car learned from minor corrections, eventually figuring out how to stay on the road by itself.
This was the first time reinforcement learning—a system that learns by trial and error—was applied to an actual car on a real road. The results were promising. Reinforcement learning had previously been successful in tasks like playing games and even controlling a nuclear reactor. But driving a real car is a much more complex challenge, involving rapid adaptation to unpredictable events on the road.
A New Path Forward
Wayve has taken this breakthrough further. Today, it tests cars in busy London traffic, training them to drive in multiple cities across the UK. Wayve’s AI successfully drove in five different cities without specific training for each one—a feat that established players like Waymo and Cruise have yet to achieve. In 2023, Wayve partnered with Microsoft to use the Azure cloud to process massive amounts of data for its AI model. This strategic move allows Wayve to scale up its AI capabilities and train its models more efficiently.
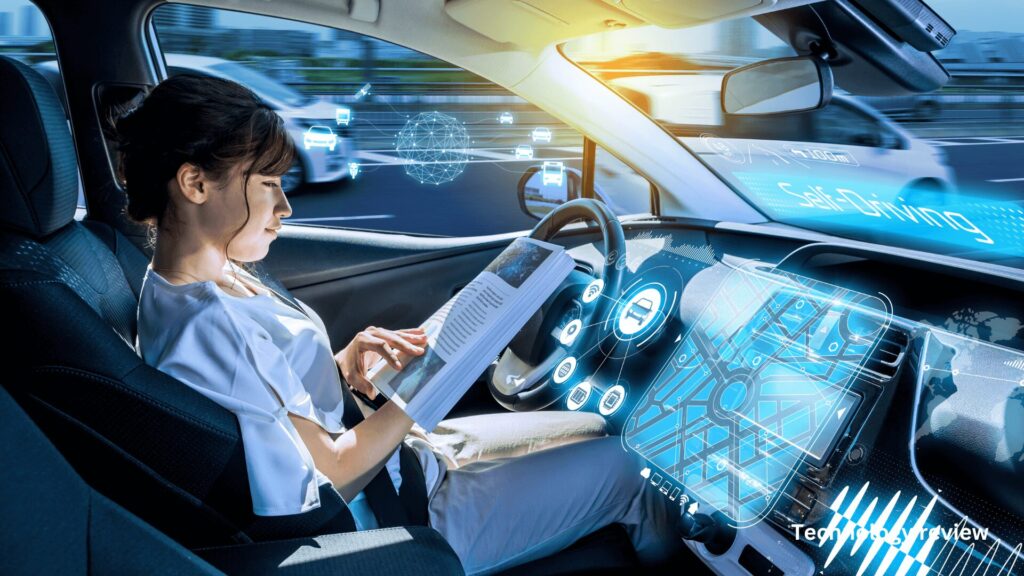
Challenges of Current Self-Driving Approaches
The current model of building autonomous cars involves separate systems for perception, decision-making, and vehicle control. Companies using this approach rely on distinct AI models for each task. It’s effective but comes with a major drawback: it requires constant updates to keep the systems working together seamlessly. Waymo and Cruise, for example, need to update each model independently. This modular system is complex and expensive to manage.
Raquel Urtasun, former head of Uber’s autonomous car team and current CEO of Waabi, notes that the current approach may never reach full autonomy. She believes the future lies in integrating these systems under a unified AI model. According to Urtasun, the old way lacks the adaptability necessary for true self-driving cars that can operate on any road, anywhere.
End-to-End Learning for Autonomous Cars
The new wave of companies, including Wayve, Waabi, and Ghost, are exploring a streamlined approach known as end-to-end learning. Instead of building a system with multiple neural networks and linking them together, they’re designing one comprehensive AI model. This model learns to turn camera or lidar input into steering and braking commands, effectively mimicking a human learning to drive.
End-to-end learning has already transformed fields like natural language processing and visual recognition. Similar models power chatbots like GPT-3 and AlphaZero’s chess strategies. These AI models learn from vast datasets, absorbing patterns and behaviors from real-world interactions. Wayve’s Kendall believes that driving can be improved in the same way, potentially reaching human-level driving ability through extensive learning.
Waabi and Ghost’s AI-First Approach
Waabi is developing its AI in a hyper-realistic driving simulation, managed by an advanced virtual driving instructor. This lets Waabi’s model practice in a safe, controlled environment, where it learns by running through millions of driving scenarios. Waabi’s reliance on simulation reduces costs and risk while allowing the AI to experience a broader range of situations.
Ghost, another AV2.0 startup, is also embracing AI-first strategies. Its technology not only steers the car but also actively learns from other drivers on the road. The system adjusts its driving based on observed human driving patterns, picking up the nuances of real-world driving. This approach aims to produce a system that’s both reactive and predictive, making it safer in complex traffic environments.
Autobrains: Solving Small Problems to Win Big
Autobrains, an Israeli startup, takes a different approach by dividing driving into thousands of smaller challenges. Instead of training one large neural network, Autobrains uses 200,000 small models, each designed to handle specific scenarios, like rain, pedestrians, or four-way stops. This granular approach simplifies the learning process, as each model only has to manage a limited set of tasks.
According to Igal Raichelgauz, CEO of Autobrains, this method reduces the system’s complexity and makes it more adaptable to new conditions. Raichelgauz believes that focusing on smaller, more manageable AI tasks allows the system to handle real-world scenarios more reliably.
The Role of HD Mapping in Self-Driving Cars
Most self-driving car companies today rely on high-definition (HD) maps. These maps offer cars precise details about street layouts, curb locations, and the position of traffic lights, aiding the AI in decision-making. However, these maps require constant updates as road conditions change frequently. Companies like Cruise develop their own HD maps for accuracy, while others purchase map data from third-party providers.
Cruise has built its San Francisco service on a base of HD maps, giving its robotaxis detailed knowledge of every street. This approach is stable but limits scalability, as new areas need mapping before the cars can safely operate there. Many argue that this is the main obstacle to global deployment of self-driving technology.
The Case Against HD Mapping
Wayve and Autobrains have chosen not to use HD maps. Wayve’s cars rely solely on sensor data, using GPS for navigation and avoiding map dependence. This decision allows Wayve’s vehicles to learn and adapt in real-time, a strategy that should make expansion to new locations faster. Wayve’s Kendall believes that while his company might take longer to reach market, its technology will ultimately scale faster once it reaches a breakthrough city.
Will AI-Centric Models Overtake Established Players?
The AI-driven approach holds great promise, but the established players remain skeptical. Mo ElShenawy, an executive at Cruise, argues that modular systems allow Cruise to implement new technology as it emerges. He believes that Cruise’s existing framework, which has been proven on the streets of San Francisco, offers a scalable solution. ElShenawy points out that while AI advancements are impressive, they may not yet be robust enough to handle the complexity of urban driving.
Cruise’s success has already led to live robotaxi services in San Francisco. The company’s cars navigate hundreds of thousands of diverse situations, proving that modular systems are dependable in limited areas. However, Kendall and others argue that scaling these systems to new cities will be much slower compared to adaptable AI-centric models.
Conclusion: The Road Ahead for Autonomous Vehicles
The new generation of autonomous vehicle companies is betting on a transformative shift. End-to-end learning, with its data-driven approach, offers the potential to unlock self-driving capabilities that could handle diverse environments without specific programming. While firms like Waymo and Cruise refine their modular systems, Wayve, Waabi, and Autobrains are pushing AI-first technologies that might lead to more adaptable vehicles.
It’s uncertain which approach will dominate, but the diversity of strategies benefits the industry as a whole. With competition heating up, advancements will come faster, and self-driving cars may become a common sight on roads worldwide sooner than we expect. Whether through modular systems or end-to-end learning, autonomous vehicles are inching closer to becoming part of our everyday lives.